Is the reign of the statistician coming to an end? The data landscape is undeniably shifting, with the rise of big data and the emergence of data scientists seemingly overshadowing the traditional role of statisticians.
Google Trends reflects this apparent shift, illustrating a surge in the popularity of "big data" while the interest in "statisticians" has waned over time. This trend isn't just a superficial observation; it touches upon a deeper conversation about the evolving demands of data analysis and the perceived skills required to navigate this new era. I recently encountered an article titled "The Risky Eclipse of Statisticians," which I found particularly insightful and thought-provoking. It articulated a sentiment that's been brewing within the data community: are statisticians being sidelined by the ascendance of data scientists?
While I'm confident in my understanding of what a statistician does the rigorous application of statistical methods, the focus on inference and hypothesis testing, the deep understanding of probability distributions the role of a data scientist feels somewhat more amorphous. What exactly differentiates a data scientist from a statistician? Is it simply a matter of branding, or do data scientists possess a fundamentally different skill set that makes them better equipped to handle the challenges of big data?
- Bam Margeras Wild Ride From Jackass Star To Recovery Journey
- Nsfw 18 Content What You Need To Know 2024 Guide
The article "The Risky Eclipse of Statisticians" dives into the core of this debate, suggesting that statisticians are indeed facing marginalization. This raises critical questions about the future of the profession and the potential consequences of undervaluing statistical rigor in the age of data deluge. If statisticians have historically been the leaders in data analysis, why the sudden need for a new breed of "data scientists" coinciding with the explosion of big data?
Consider the sheer scale of the data science community online. The datascience community on Reddit, for example, boasts 1.3 million subscribers a vibrant space for professionals to engage in discussions and debates about data science. This active online presence underscores the growing interest in and demand for data science expertise. It's a stark contrast to the often more academic and less visible world of statistics.
This shift in focus is mirrored in discussions across various online platforms. Even within the hackernews community (78k subscribers), a mirror of Hacker News' best submissions, you'll find threads pondering the evolving role of statisticians in the data-driven world. Are statisticians being left behind, or are they simply adapting to the changing landscape under a different title?
The real question lies in whether the core principles of statistical thinking are being diluted in the rush to embrace big data technologies. Is the emphasis on predictive modeling and machine learning overshadowing the importance of understanding the underlying statistical assumptions and limitations of these methods? Are we prioritizing speed and scalability over accuracy and interpretability?
My own interest in this topic stems, in part, from a personal connection. I had a relative who possessed a remarkable aptitude for statistics. Their ability to extract meaningful insights from complex data sets was truly impressive. It makes me wonder, what would they think of this current debate? Would they embrace the rise of data science, or would they lament the potential loss of statistical rigor?
The perceived marginalization of statisticians isn't just an abstract concern; it has real-world implications. Consider the field of medicine, where statistical illiteracy can have devastating consequences. Heaths review highlights this issue, criticizing the "devastating dissection of the statistical illiteracy of doctors," while simultaneously arguing that the real problem lies in the "devastating biomedical ignorance of statisticians." This raises a crucial point: effective data analysis requires not only statistical expertise but also a deep understanding of the specific domain in which the data is being applied. Why should a doctor need to know how to calculate the chance of breast cancer in a patient with a positive screening mammogram? The answer underscores the vital need for statistically sound reasoning in medical decision-making.
Examples abound of how this tension plays out. Articles by Ritika Trikha on Muck Rack offer a window into this evolving world. Finding Trikha's email address, contact information, LinkedIn, Twitter, and other social media details can provide a professional lens on the matter. Furthermore, research such as "Statistical thinking and its impact on operational performance in manufacturing companies: An empirical study" by Fabiane Letcia Lizarelli, Jiju Antony, and Jos Carlos Toledo, underscores the enduring value of statistical principles in practical applications.
Companies like Gamestop, Moderna, Pfizer, Johnson & Johnson, AstraZeneca, Walgreens, Best Buy, Novavax, SpaceX, and Tesla are all grappling with massive amounts of data. Their ability to leverage this data effectively depends on having professionals who can not only build predictive models but also critically evaluate the results and ensure that they are statistically sound. The future hinges on bridging the gap between traditional statistical methods and the demands of big data.
Agarwal, in "The Information Systems Identity Crisis," touches on similar themes of how evolving technological landscapes impact professional roles and identities. The key takeaway is that adaptability and continuous learning are essential for professionals to remain relevant in a rapidly changing world.
Even in risk management, the principles of statistics are paramount. Eclipse Risk Management (ERisk) emphasizes the importance of traceability in risk assessment results and decision-making. Without a solid understanding of statistical principles, it's impossible to accurately assess and mitigate risks.
The decline in the popularity of statisticians, as suggested by Google Trends and articles like "The Risky Eclipse of Statisticians," should serve as a wake-up call. It's time to re-evaluate the way we train and educate data professionals, ensuring that they possess a strong foundation in statistical thinking. It's not about choosing between statisticians and data scientists; it's about fostering collaboration and integration, leveraging the strengths of both disciplines to unlock the full potential of data.
We need to promote data literacy across all sectors, empowering individuals to critically evaluate data and make informed decisions. This includes educating doctors on the importance of statistical reasoning, as well as equipping business leaders with the skills to interpret data and make data-driven decisions. Only then can we truly harness the power of data to improve our lives and solve the world's most pressing challenges.
The narrative isn't just about numbers; it extends to broader societal trends, as reflected in topics circulating online: "The history of aliens in film," "Exploding the nuclear family," "If you're feeling unmotivated today, watch this video," "Here's what breaking up does to your brain," "What alien hunters plan to buy with $100 million." These seemingly disparate topics highlight the public's diverse interests and the need for data professionals who can analyze and interpret data from a wide range of sources.
The debate surrounding the "risky eclipse of statisticians" is far from over. It's a conversation that will continue to evolve as technology advances and the demand for data-driven insights grows. Ultimately, the key to success lies in embracing a holistic approach to data analysis, one that values both statistical rigor and technological innovation.
- 2025 Indian Streaming Scene Mustwatch Hindi Web Series
- Mustsee Indian Erotic Web Series Your Ultimate Guide
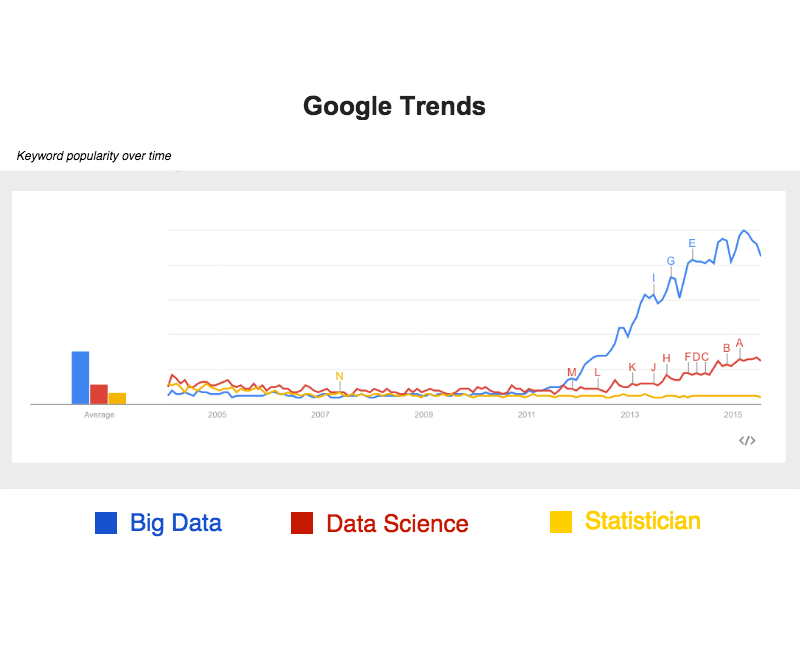
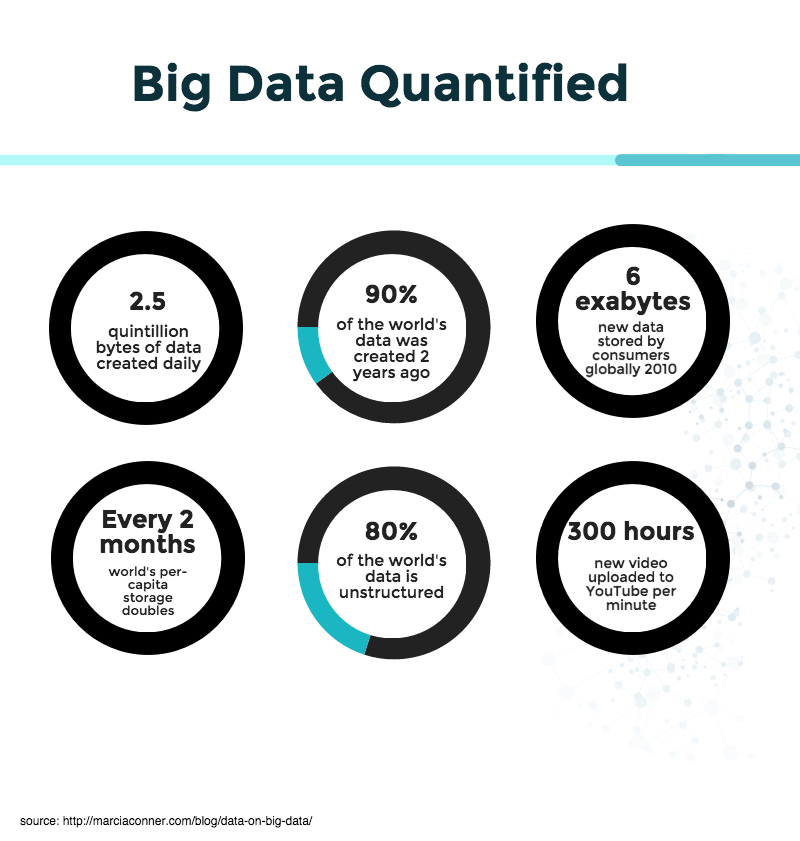
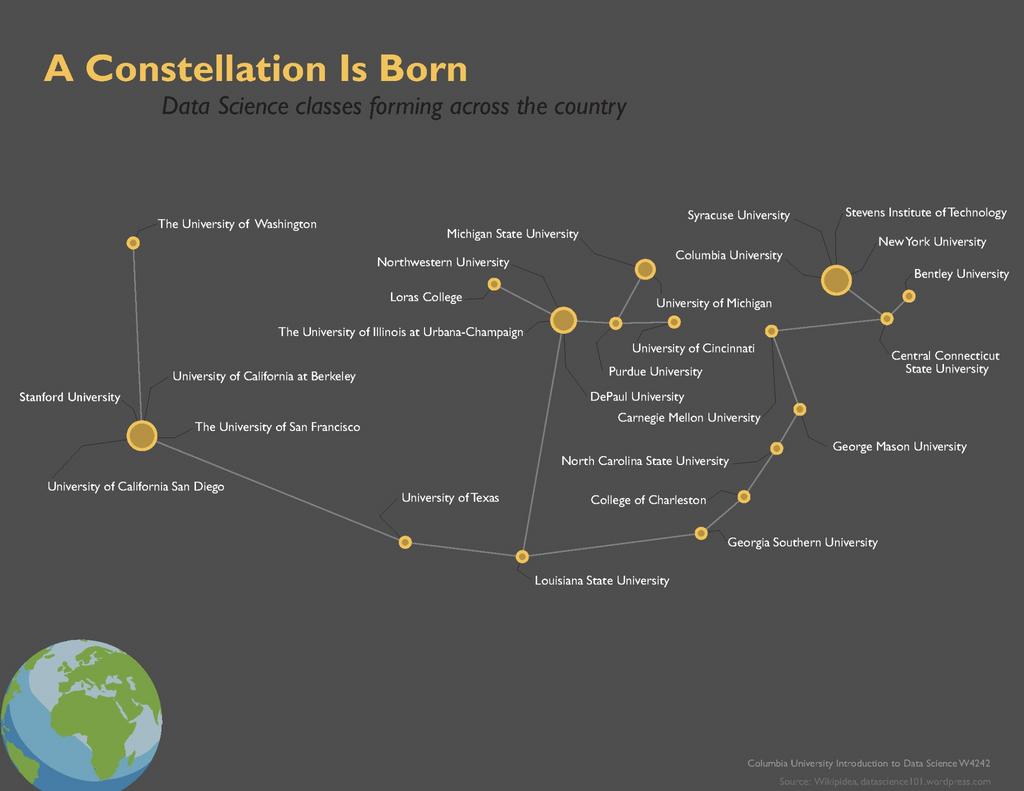